
Zhou, K., Wei, R., Xu, Z., Zhang, Q., Zhang, G.: An air combat decision learning system based on a brain-like cognitive mechanism.
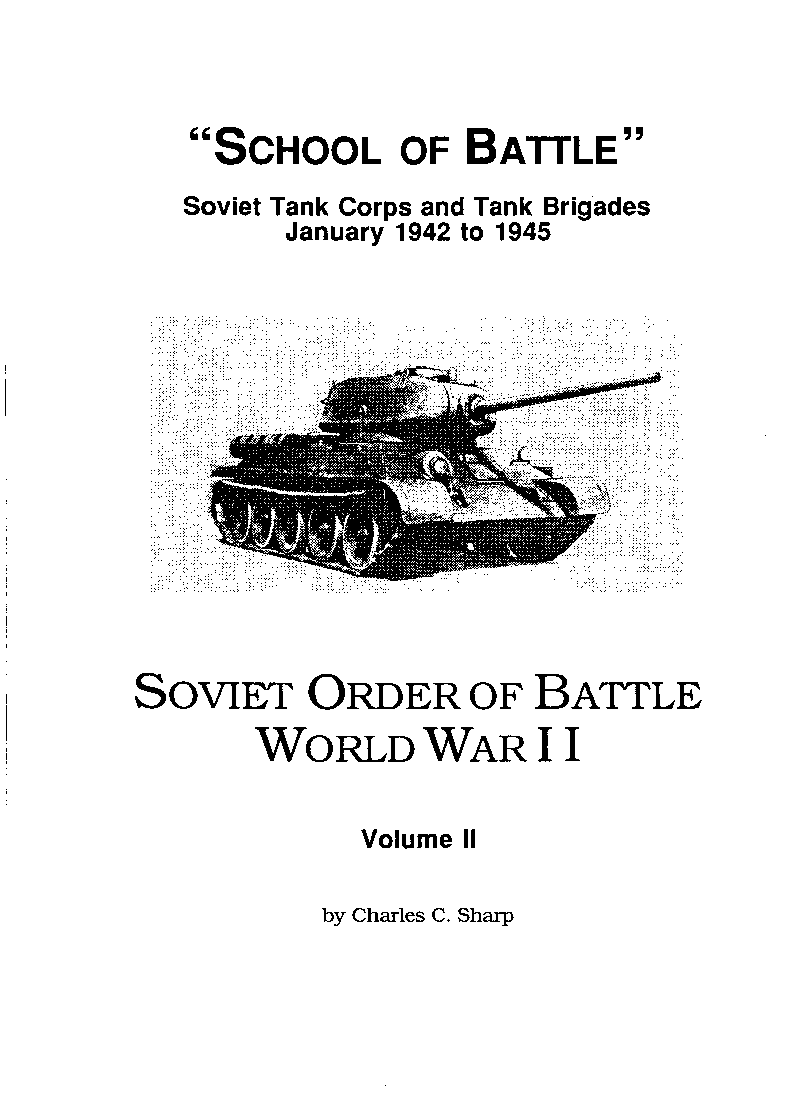
Zhang, L.P., Wei, R.X., Li, X.: Autonomous Tactical Decision-Making of UCAVs in Air Combat. Zhou, K., Wei, R., Zhang, Q., Xu, Z.: Learning System for air combat decision inspired by cognitive mechanisms of the brain. Huang, “Air combat situation assessment for UAV based on improved decision tree”, pp. Virtanen, K., Karelahti, J., Raivio, T.: Modeling air combat by a moving horizon influence diagram game. Virtanen, K., Raivio, T., Hmlinen, R.P.: Modeling pilot”s sequential maneuvering decisions by a multistage influence diagram. Kai, Virtanen, Tuomas, Raivio, Raimo, P., H-aring, m-aring, l-aring, and inen, “Decision Theoretical Approach to Pilot Simulation”, Journal of Aircraft, 36(4), 632 (1999) IEEE Transactions on Systems Man and Cybernetics - Part A Systems and Humans 40(5), 1057–1070 (2010) Poropudas, J., Virtanen, K.: Game-theoretic validation and analysis of air combat simulation models. Proceedings of the Institution of Mechanical Engineers 233(16), 5943–5991 (2019) Liu (2019) “UAV Air Combat Autonomous Maneuver Decision Based on DDPG Algorithm”, IEEE 15th International Conference on Control and Automation (ICCA), ĭong, Y., Ai, J., Liu, J.: Guidance and control for own aircraft in the autonomous air combat, A historical review and future prospects.

Newman, D.G., Callister, R.: Analysis of the Gz environment during air combat maneuvering in the F/A-18 fighter aircraft. Kim, C.S., Ji, C.H., Kim, B.S.: Development of a control law to improve the handling qualities for short-range air-to-air combat maneuvers. Wu, Z., Wu, H., Li, H., Wang, Z.: Improving maneuver strategy in air combat by alternate freeze games with a deep reinforcement learning algorithm. Karimi, I., Pourtakdoust, S.H.: Optimal maneuver-based motion planning over terrain and threats using a dynamic hybrid PSO algorithm. Lee, “Genetic Fuzzy based Artificial Intelligence for Unmanned Combat AerialVehicle Control in Simulated Air Combat Missions,” Journal of Defense Management, 06(1) (2016) The fuzzy reasoning method provides a new solution on improving the UCAV self-decision ability.Įrnest, N., D. Finally, simulation results show that the fuzzy reasoning method could guide UCAV to make more targeted maneuver decisions according to the real-time combat situation. As an auxiliary means of maneuver decision-making, the enemy aircraft position prediction model integrating decision-maneuver, sequence-maneuver, and inertial-maneuver is proposed. The advantage factors representing the air combat situation are input into the fuzzy reasoning machine to adaptively adjust the weight of each factor in the advantage function. Secondly, the fuzzy rules are used to evaluate the air combat situation. Firstly, four advantage factors of angle, distance, speed, and height are established from the air combat scene. In view of the high dynamic, uncertain and time-varying characteristics of unmanned combat aerial vehicle (UCAV) air combat situation, a maneuver decision method based on fuzzy reasoning is proposed.
