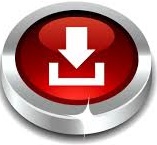
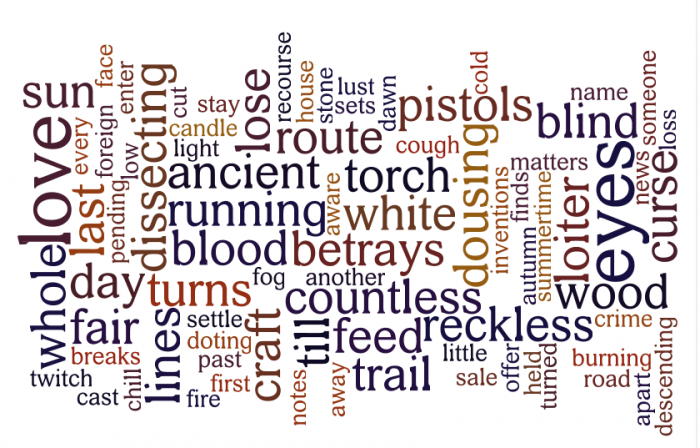
Semantic textual similarity is measured by way of a distance metric, typically cosine similarity. For example, we would expect the words “orange” and “apple” to be close, while, say, “house” or “space ship” should be further away from the pair. In the high-dimensional Word2Vec embedding space, similar words lie close to each other. These vectors are dense, meaning that they consist of mostly floating point values, rather than zeros. Both train a shallow neural net to represent words as feature vectors of variable length (typically 300). There are two main implementations of Word2Vec ( CBOW and skip-gram). In 2013, a team led by NLP researcher Tomáš Mikolov came up with the Word2Vec method, which could represent the semantic and syntactic properties of words through “word embeddings.” Word2Vec follows the idea that the meaning of words lies in their distributional properties - the contexts in which a word is used. The sparse, count-based methods we saw above do not account for the meaning of the words or phrases that our system processes. In short, we would understand its semantics.
Vectorize text how to#
As speakers of a language, we might understand what a word means and how to use it in a sentence. Words are more than just a collection of letters. Let’s now look at a more recent encoding technique that aims to capture not just the lexical but also the semantic properties of words. Semantic search systems use them for quick document retrieval. It also dampens the effect of having many occurrences of a word in a document.īecause BoW methods will produce long vectors that contain many zeros, they’re often called “sparse.” In addition to being language-independent, sparse vectors are quick to compute and compare. It is an improvement over TF-IDF, in that it takes into account the length of the document.

BM25 was introduced to address this and other issues. For example, it does not address the fact that, in short documents, even just a single mention of a word might mean that the term is highly relevant. While more sophisticated than the simple BoW approach, TF-IDF has some shortcomings. In that case, it helps characterize the document within the corpus and as such receives a higher value in the vector.
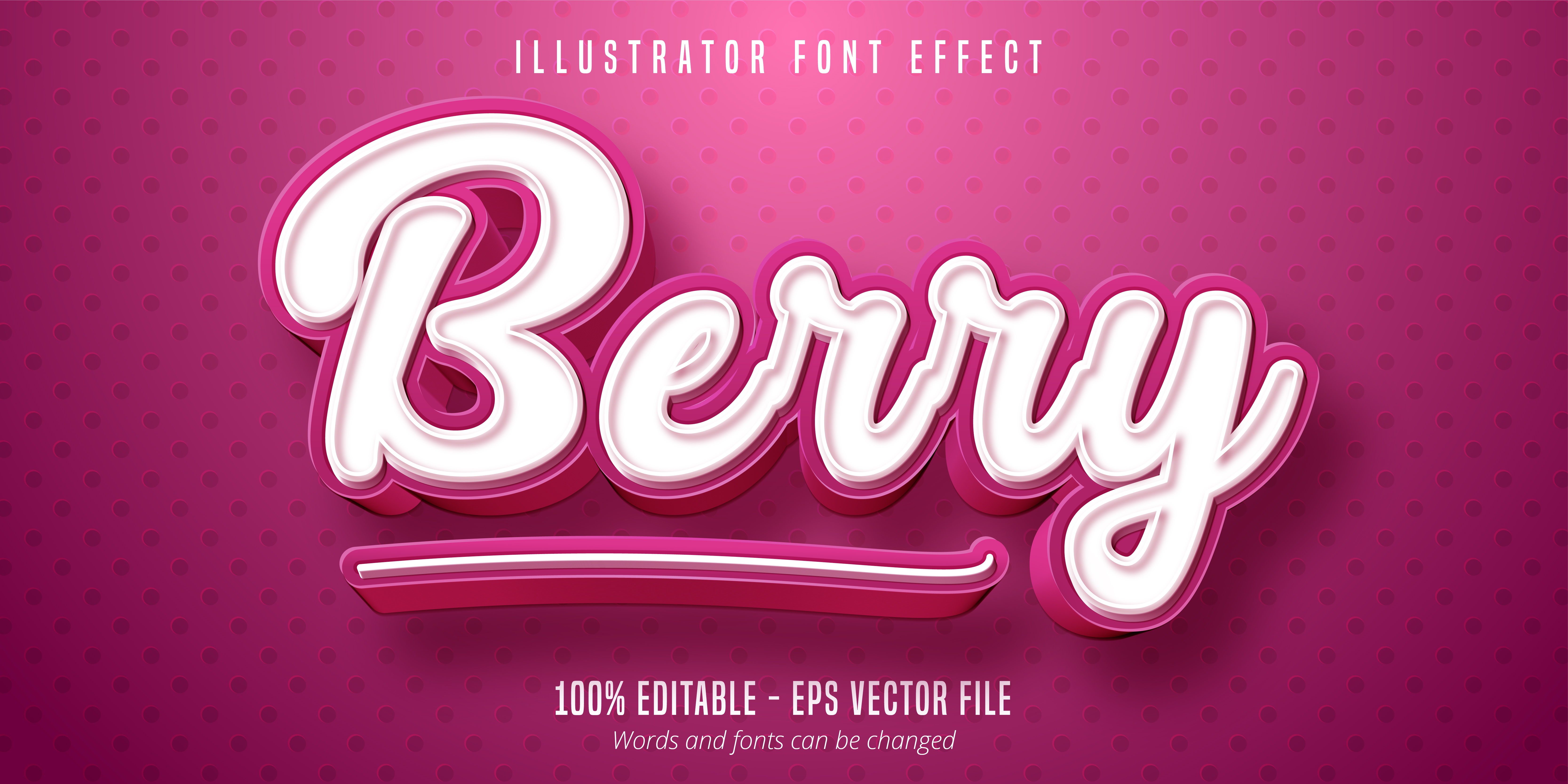
If it occurs in just a few documents, however, it is considered a distinctive term. If “oranges” occurs in many documents, then it is not a very significant term and is given a lower weighting in the TF-IDF text vector. TF-IDF will look at all the other documents in the corpus. Think of a document that mentions the word “oranges” with high frequency. To that end, TF-IDF measures the frequency of a word in a text against its overall frequency in the corpus. Weighted BoW text vectorization techniques like TF-IDF (short for “term frequency-inverse document frequency), on the other hand, attempt to give higher relevance scores to words that occur in fewer documents within the corpus. The vector’s values represent the frequency with which each word appears in a given text passage: A BoW vector has the length of the entire vocabulary - that is, the set of unique words in the corpus. One of the simplest vectorization methods for text is a bag-of-words (BoW) representation. They offer a uniform format that computers can easily process. For the purpose of input representation, it is simply a succession of values, with the number of values representing the vector’s “dimensionality.” Vector representations contain information about the qualities of an input object. In programming, a vector is a data structure that is similar to a list or an array. Count-Based Text Vectorization: Simple Beginnings Finally, we will look at the recent and exciting trend of vector databases. We will discuss how Transformer-based language models have brought deep semantics to text vectorization, and what that means for modern search systems. We’ll briefly look at traditional count-based methods before moving on to Word2Vec embeddings and BERT’s high-dimensional vectors.
Vectorize text full#
In this post, we’ll track the history of text vectorization in machine learning to develop a full understanding of the modern techniques. But what exactly are those vectors, and how can you use them in your own applications? Other data types, like images, sound, and videos, may be encoded as vectors as well. In natural language processing (NLP), we often talk about text vectorization - representing words, sentences, or even larger units of text as vectors (or “vector embeddings”). For as long as we have had computers, there has been the question of how to represent data in a way that machines can work with.
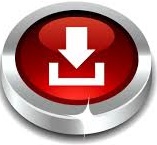